Until very recently, most AI pundits believed that the dominant use of AI would be to take over repetitive human tasks, and creative pursuits should be left to humans. The emergence of Generative AI and its exponentially advancing capabilities have proved that these claims were so wrong! The magical capabilities of ChatGPT would put most writers to shame.
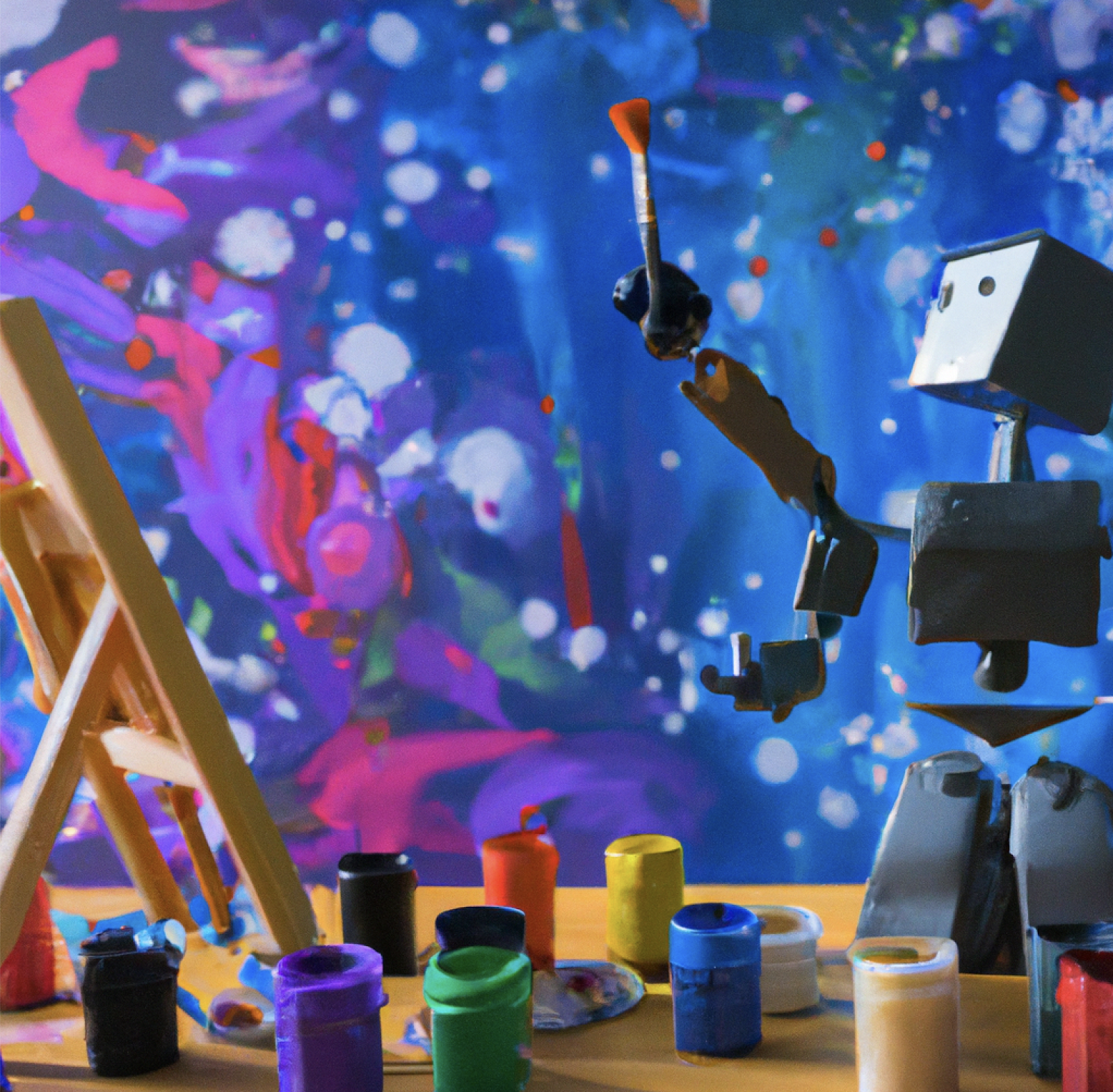
Generative AI is a powerful subset of artificial intelligence that allows machines to create and generate novel outputs such as images, music, and text. This incredible breakthrough in machine learning that allows machines to generate completely new artifacts is transforming the way we approach many different problems, from image recognition to natural language processing. Generative AI is capable of synthesizing new data that is not based on pre-existing data inputs, making it a valuable tool for generating new ideas and solutions.
At its core, generative AI is based on the principles of deep learning, a type of machine learning that is designed to simulate the workings of the human brain. Generative models rely on neural networks, which are algorithms that process information in a way that is similar to the way neurons in the human brain work. These neural networks can learn from a large set of data and use that knowledge to generate new outputs that resemble the original data. Due to the advances in cheap computational power, and enormous amounts of data available, thanks to the Internet, we can now train extremely complicated neural networks. E.g. GPT3, the third-generation Generative Pre-trained Transformer, that ChatGPT (and KNO) is derived from contains 175 “billion” parameters.
How does Generative AI work?
Generative AI models are built using complex mathematical algorithms that allow the machine to learn from patterns and predict new data. The most common approach is to use a neural network, which is made up of many interconnected layers of artificial neurons. Each neuron in the network is capable of receiving input from multiple sources and processing that input to produce an output. The connections between neurons are weighted, which allows the network to learn from experience and adjust its predictions accordingly.

Generative AI models use a variety of techniques to generate new data. One of the most common approaches is the Generative Adversarial Network (GAN). GANs consist of two neural networks: a generator network and a discriminator network. The generator network generates new data based on random inputs, while the discriminator network determines whether the generated data is real or fake. The two networks are trained together, with the generator trying to generate data that can fool the discriminator, and the discriminator tries to correctly identify real data from fake data. Over time, the generator network becomes better at generating data that is indistinguishable from real data.
Another common approach is the Variational Autoencoder (VAE). VAEs use a different method to generate new data. Rather than learning to generate data from scratch, VAEs learn to represent existing data in a more compact form. This representation is called a latent space. The VAE can then use the latent space to generate new data that is similar to the original data.
What can Generative Models do?
There are several different types of generative AI models that are used to generate different types of data. Some of the most popular models include:
- Image Generative Models are used to generate images. Examples of image generative models include Deep Convolutional Generative Adversarial Networks (DCGANs) and Variational Autoencoders (VAEs) powering tools like Dall-E 2 and Stable Diffusion.
- Text Generative Models are used to generate new text. Examples of text generative models include Recurrent Neural Networks (RNNs), Long Short-Term Memory Networks (LSTMs), and Transformers. The widely popular ChatGPT and Codex fall under this category. This is also what powers the conversational engine at KNO.
- Music Generative Models are used to generate new music. Examples of music generative models include MusicVAE and LSTM Networks being used by companies such as Avia.
- Video Generative Models are used to generate videos. Examples of video generative models include Deep Video Networks (DVNs) and Variational Autoencoder Recurrent Networks (VARNs) and used by companies like Descript and Synthesia.
So what is the big deal?
Generative AI has the potential to improve human performance in many different areas, including
- Creativity - it can help artists, musicians, and writers explore new creative ideas and generate new works of art. By providing new and unexpected inputs, generative AI can help creatives break out of their creative ruts and come up with new and exciting works. I believe this will usher in a new renaissance in the arts.
- Design - it can help designers create new designs and prototypes quickly and efficiently. By automating the design process, generative AI can save designers time and resources, allowing them to focus on the more creative aspects of the design process. We have already seen some fine examples of man-human collaboration in Zaha Hadid’s masterpiece.
- Healthcare - GenAI is helping doctors and researchers analyze medical data and develop new treatments for diseases by designing new proteins which might lead to a future where few diseases are without a cure.
- Business - by analyzing large amounts of data, GenAI can develop new strategies for growth and innovation. By generating new ideas and possibilities, generative AI can help businesses stay ahead of the competition and adapt to changing market conditions.
- Education - will be one area most impacted by Gen AI. It can help educators develop new teaching materials and can finally offer really personalized learning for individual students.
By providing new inputs and generating new possibilities, this technology can help us achieve new levels of creativity, efficiency, and effectiveness.
At Alltius, we discover new use cases every day, and frankly, I am personally amazed at how many things KNO can do well without explicit training. In fact, we are so knee-deep into Generative AI, that almost every part of our business uses some aspect of it, from forming the bedrock of our core learning platform, writing code, and all the way to creating marketing campaigns, including some parts of this article.
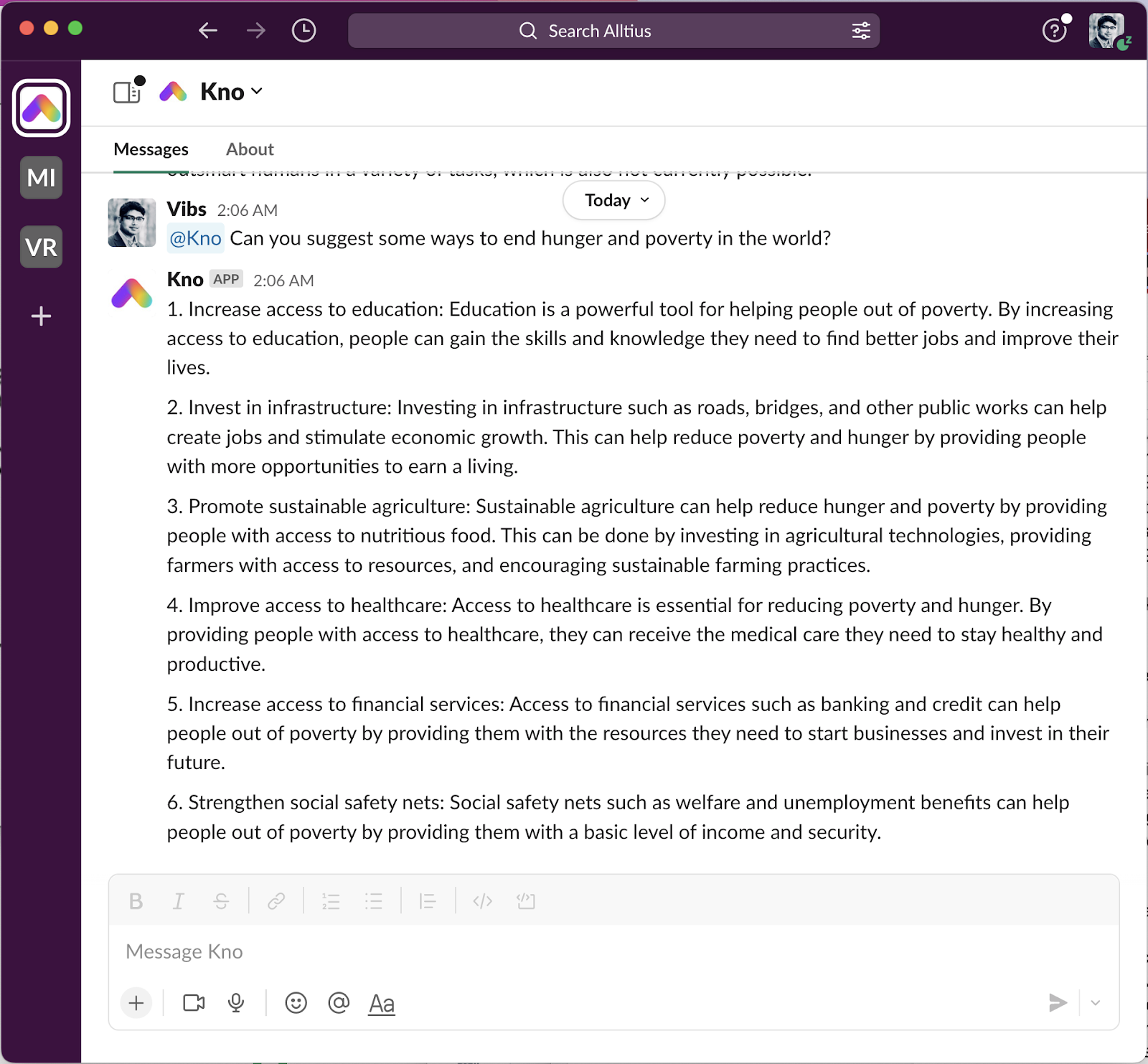
All is not great in (Gen) AI land
While generative AI models have many strengths, there are also some weaknesses associated with generative AI models. One of the biggest challenges is the potential for bias in the generated data. This can be especially problematic in fields such as healthcare, where bias can have serious consequences. Another challenge is the difficulty in interpreting and understanding the generated data, which can make it difficult to use the models in practical applications.
The second biggest challenge is that all the enthusiasm about GenAI might be misplaced. E.g. large language models that power tools like ChatGPT are very limited in their understanding of the world. Very simply, LLM likes GPT3 to predict the next token (or word) that should appear given a set of preceding tokens. Their power comes from all the (Internet) scale data that their models are trained on, and they can do amazing things, but very simply they are probabilistic machines. They do not have intelligence as we define intelligence, i.e. they do not understand the structure of the world, or construct abstractions and knowledge graphs. Not surprisingly, they are prone to hallucinations. This might be OK for cases such as writing a fictional piece, but might not pass muster while writing credibly about a recent event.
Thirdly, Gen AI is already having a significant impact on the labor market, and this impact is likely to continue in the future. As machines become more adept at generating new data and ideas, it is likely that many jobs will become automated. This is already happening in fields such as manufacturing and logistics, where robots are being used to perform tasks that were previously done by humans. However, as pointed out earlier generative AI also has the potential to create new jobs in fields such as creative arts and healthcare.
Another larger concern about Generative AI is our lack of understanding of its power. I am amazed by its power, and it doesn’t do anything short of magic. And while magic creates awe, it can very soon turn to fear. What we don’t understand, we can’t control. While I do not believe that AI would become sentient anytime soon, I also couldn’t imagine even in my wildest imagination 4-5 years back that we would have KNO-like systems in the year 2022.
What lies ahead?
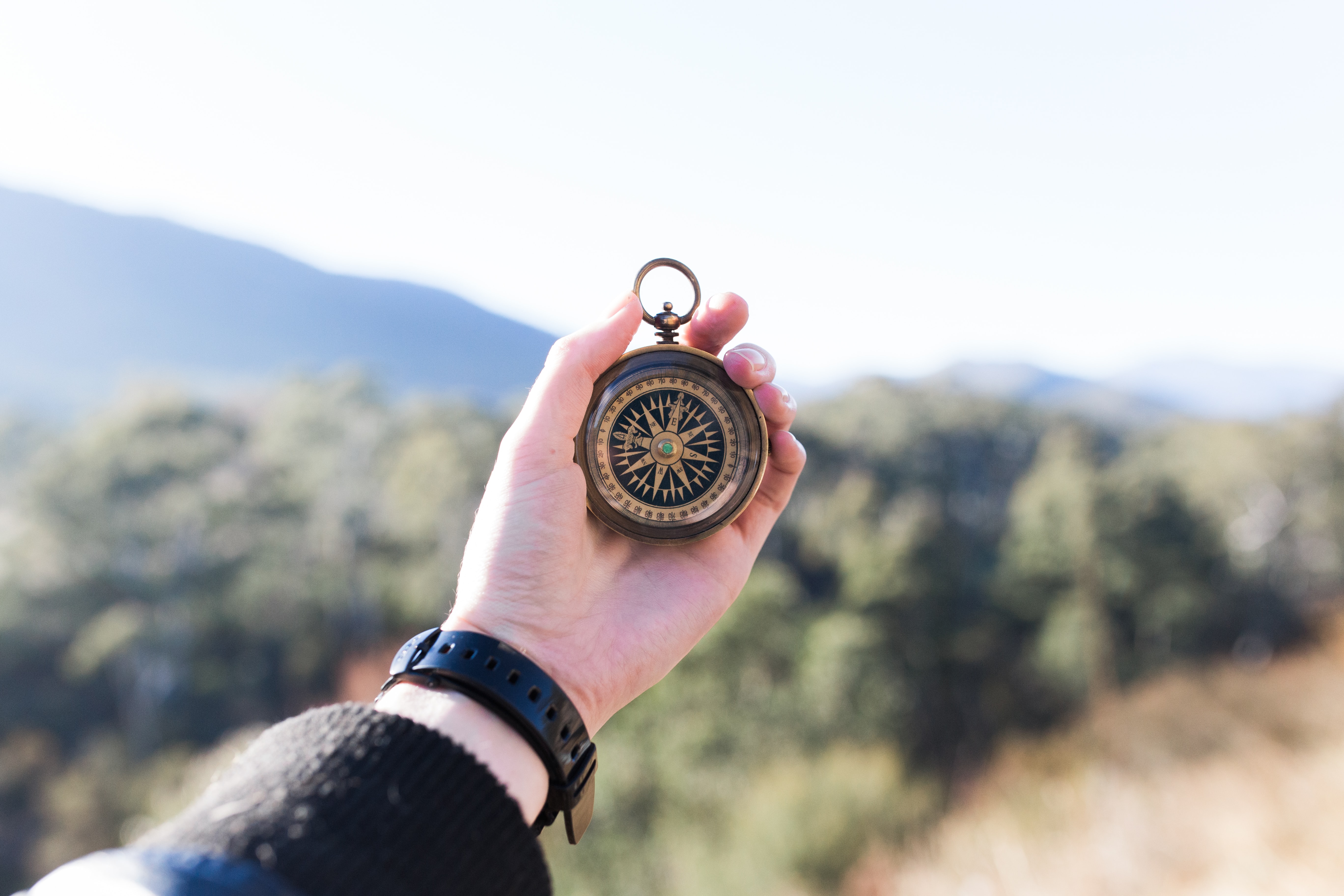
In my mind, Generative AI reflects an inflection point, probably as or more powerful as fire or the Internet. It will become an indispensable tool for solving complex problems and exploring new creative ideas, and it will significantly hasten mankind’s pursuit. As someone who has spent over two decades working on AI, it seems like I am living my childhood dream. This stuff is real, and I am extremely optimistic about the positive impact it will have in the near future.
But eventually, will it lead to the creation of a utopian world with extremely high efficiency such that hunger and poverty are completely eliminated, or will it lead to a world where humans are slaves to our AI overlords?
Only time will tell, but it will be one hell of a ride!